Understanding the Risks in Structured Capital Models
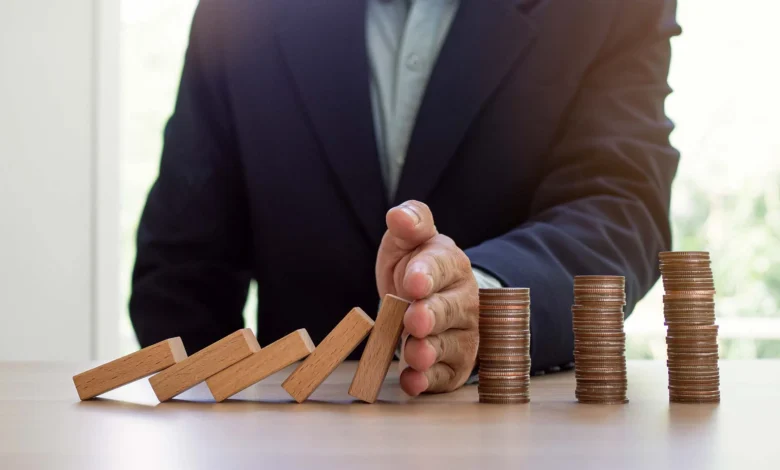
Optimizing capital allocation and evaluating financial risk depends on organized capital models. They provide a methodical methodology to assess an institution’s degree of exposure to several market, credit, and operational hazards. These models provide a variety of difficulties that could greatly influence decision-making even if they provide exact quantitative information. Major financial crises resulting from misjudgments within these systems expose the complexity buried under their surface. Whether handling big-scale institutional portfolios or private trading techniques, knowledge of the hazards included in structured capital models is very vital. Dealing with these risks means not only improving the models but also keeping a critical viewpoint on their constraints and adjusting to a changing financial scene.
Unspoken Weaknesses in Model Assumptions
Models of structured capital are only as trustworthy as the presumptions upon which they are based. Crucially, these presumptions support factors like liquidity dynamics, market behavior, and asset correlations. The outputs of the model become erratic when these fundamental assumptions differ from reality, therefore producing erroneous risk evaluations. One main issue is many models’ unchanging character. Affected by geopolitical events, governmental changes, and technology developments, financial markets are by nature vibrant.
Models that fail to change with the times provide outcomes that understate possible threats. For example, presumptions about consistent asset correlations can fall apart during market stress, increasing losses. Structured capital models also usually assume normal market distributions. This ignores the occurrence of tail-risk events, severe, erratic situations capable of causing major financial loss.
Data Quality and Affecting Model Integrity
Any disciplined capital model starts with accurate data. Incorrect or lacking data compromises the accuracy of risk estimations and assessments. The quality and relevancy of the data entering a model determine not just its accuracy but also its quantity. Inaccurate assessment of exposure and outcome prediction might follow from poor data governance. Many times, data gaps result from models depending on past data that miss newly arising hazards. For instance, modeling future events using pre-crisis data ignores fundamental changes in the financial system.
Insufficient data cleansing and validation raise the possibility of mistakes that affect risk assessments even further. Moreover, organized capital models need strong procedures for maintaining and upgrading databases. The amount of data increases rapidly as more complicated financial products develop.
Operational Risk Found in Model Execution
Implementing structured capital models adds even another degree of risk because of their operational complexity. Turning theoretical ideas into useful systems calls for several steps, each prone to mistakes and inefficiency. From programming errors to misread calculations, implementation problems affect risk forecast accuracy. One main cause of operational risk in human intervention is that Decision-makers, depending on model outputs, could overestimate their accuracy without fully appreciating the limits of the model. Prop trading firms face heightened risks due to their reliance on rapid and precise model execution.
In these environments, even small discrepancies in algorithmic systems can trigger cascading losses across entire portfolios. One mistake in an algorithmic trading system might cause whole portfolios to suffer from cascading losses. Furthermore, very important in reducing operational risks are internal governance systems.
Stress Testing and the delusion of readiness
One of the most important tools for evaluating how well-organized capital models hold under demanding circumstances is stress testing. However, it might provide a false sense of security if it is poorly thought out or executed. The situations selected and the degree of the research carried out determine the validity of stress testing. Many stress assessments center on historical events, supposing future crises would reflect previous trends. This method ignores new hazards and black swan events—unexpected disturbances against accepted modeling methods.
Dealing with Responsibility
Management of the risks in structured capital models depends on good model governance. It covers the methods for model building, validation, and continuous observation. Weak governance structures allow unbridled assumptions, mismatched incentives, and poor control, thus generating major risks. The absence of responsibility in model decision-making raises among the most urgent issues. The absence of clear ownership results in missed mistakes and delayed required fixes.
The complexity of structured capital models, which can include many departments with different goals, aggravates this problem. Well-defined responsibilities and open channels of communication assist to avoid inconsistent model implementation. Regulatory compliance also gives model governance even another degree of complication. Financial authorities want institutions to have auditable and open risk models.
Conclusion
Modern financial risk management still relies on structured capital models, which are still very essential, but their efficacy depends on a thorough knowledge of the hazards they include. Essential actions to minimize these weaknesses include strengthening data integrity, enhancing operational procedures, and supporting strong governance. Financial institutions may negotiate the complexity of capital modeling with more confidence and resilience by realizing the limits and welcoming ongoing progress.